Metrics that Matter: Taking action on data
This article was written by Dorian Johnson, Head of Product at HeapAs we become increasingly data driven, we are inundated with countless sources of data. There’s attribution data, email data, behavioral data, support data, CRM data, and more. As data volume increases, it’s important to keep in mind why numbers are useful: KPIs and success metrics are only good when they are actionable.At Heap, we’ve found our customers' needs are increasingly complex. As we’ve iterated on our product, we’ve found there are four main success criteria that drive our decisions:
- adoption
- usability
- value
- vision
These criteria are ordered in increasing importance, but decreasing ease of measurement. This makes it easy to over-index on the easily measurable in destructive ways, so we should try to measure the intangibles, like adhering to our vision. This is a non-ideal default that requires active effort and frequent iteration if you’re going to be data driven organization.
Adoption — a first order approximation of whether or not something is discoverable enough. Be careful not to over-index on this! It’s easy to measure people trying a new feature out, but that doesn’t necessarily mean your feature is good or useful.
Usability - another first order metric that measures quality by measuring completion rates through specific product flows. This should also be measured with a grain of salt, a new intuitive flow could be covering for a product defect, etc.
Value - This is where it gets murkier, but also closer to what you care about.
Vision - This is the most important thing. Your product should provide far more value in the future than it does now in order for your company to grow and compete, and it’s not worth being short-sighted regarding feature development if it compromises your long-term vision. On the other hand, your vision should be constantly changing based on what you see in the field. There should be a push-pull relationship between these two aspects, and it’s useful to have human advocates in each camp, and some shared data.
Before each feature launch we set a goal for each of these metrics:
Adoption Rate: n% of our customers who fall into segment x will engage with this feature within the next three months.
Usability: n% of users who start engaging with our product will successfully complete the flow.
Value: n% of our users will engage with this feature on a monthly/weekly basisVision: This feature enables customers to do x, so we expect to see a n% increase in y
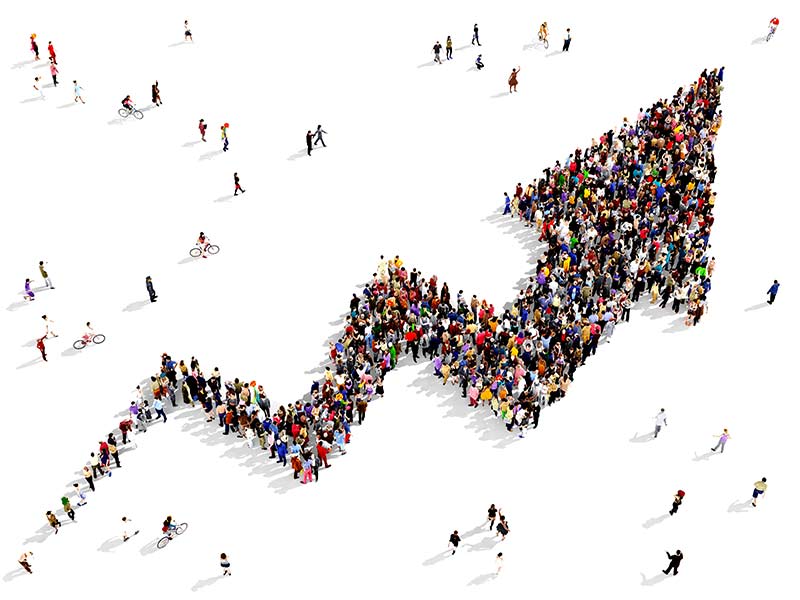
These goals help us communicate with different teams and give everyone insight into what we are aiming to accomplish. They also form a baseline that allows us to rapidly iterate on our entire product process. It’s important to note the product doesn’t exist in isolation - it’s part of a wider customer experience. Take the time to look at the entire customer story, from marketing to sales to engagement, to really understand the big picture. We ensure all of the data in our entire ecosystem is collocated so we can understand how each piece works together, and what to iterate on.Once we introduce a new feature, we make sure to track our progress against these goals so we can take any action needed as soon as possible.
Acting on our Metrics
Adoption RatesIf our adoption rate isn’t meeting expectations we ask two questions: Are we messaging the value correctly?Is it discoverable? We combine marketing and behavioral data to understand messaging vs. discoverability. By measuring traffic from marketing emails, feature engagement, and visits to our product from users who haven’t engaged with the feature we can determine the root issue. If users are reading the email and visiting the site, but not interacting with feature it indicates people want to use it, but it’s difficult to find or hard to use. We can iterate on the product immediately. If people read the email but don’t visit, it suggests our messaging is off - we didn’t explain the value is a way that excites our customers and our product marketing team can iterate on their messaging.
Similarly, we can combine CRM and behavioral data to understand who is using the feature - are Ecommerce customers using a feature and not SaaS customers? This data helps understand which customers we should speak with to understand how we can improve. We can then measure the effectiveness of each change we make to ensure we’re growing in the right direction. Each missed goal sheds light where we can improve and how to better address our customers’ needs next time.
Usability
After user testing, betas, and internal QA, releasing your product to your entire customer base can still produce unexpected results. Mapping out the steps a user needs to take in order to successfully engage with a part of your product will reveal if users get stuck and where it happens. If you look at your funnel and see drop off at step 3, you know exactly what part of the product to iterate on. It’s also important to look at your conversion rates in correlation with other parts of the customer experience. Do users have high completion rates, but 30% send an email to support? This signifies the flow is not intuitive and it still needs improvement. Are customers less likely to get stuck if they’ve read the documentation? Incorporating tooltips may help customers find success in a complicated flow.
Value
Measuring sustained engagement is the clearest indicator of value, and arguably the most important metric to track. High engagement means a job well done! The feature is valuable to your end users, and they’re incorporating it into their flows repeatedly. If you have low rates of repeat engagement, it’s important to understand which users are not engaged and why. Are low NPS scores correlated with using a particular part of your product? Combining CRM and engagement data helps discover if there is a particular persona who isn’t getting value from the feature. It can also be an indicator that the feature is hard to use, resulting in customers avoiding it. Speaking with these customers helps develop an understanding the mismatch between their expectations and the way the feature works.
Vision
Setting vision-based goals is the most variable and difficult, but it is what ultimately drives the product forward. Every feature we develop should fit together, and improve customer happiness and engagement across the product. When a feature moves us closer to our goals we see upticks in retention correlated with feature engagement, wider adoption across a company, or an increase in new users. We recently used this framework to analyze the success of our granular permissions. We had adoption goals and usability goals around engagement (80% of enterprise customers using permissions within the first quarter of its release). We also created quantifiable metrics around value and vision by combining Clearbit, Salesforce, and behavioral data in Heap to determine growth in active teammates and users invited to the account and increased breadth of titles on the account. These help quantify internal adoption (value) and bringing data-driven decisions to the entire organization (vision)......This may seem like a lot to digest, but it's all about using the right data to monitor and improve on each step of the process. Once you've zero'ed in on the numbers that tie to your problem, making actionable decisions is easier than ever.
For more on how to put tracking and analytics data to work for your business, visit heapanalytics.com